Key Takeaways
- AI price optimization means dynamically changing the price for a given service based on the customer’s behavioral and statistical data received from the system all in real time, theyby increasing the profit margin.
- These data may include your system’s historical pricing data, your competitors data for the specific service, identifying the demand in the market and the current trend based on the geographical region for that specific service.
- The algorithm should be capable on predicting the real time demand in the market, identify the threshold price for the maximing the revenue, and adjust the prices accordingly for the specific service that benefits both the system owner and the customer.
- The well-known companies like Uber and Lyft use an AI price optimization model to adjust the prices in real time based on the real-time demand in that specific georegion.
The transportation & logistics industries are always researching and exploring for methods to reduce their expenses and maximize their profits. The best to achieve this is by using AI price optimization, also known as dynamic pricing.
It’s a classic profit generating method. Showcasing on how companies execute AI algorithms to dynamically change the price for a specific service based on the real time data received without manually changingit. That helps in maximizing their revenue and inturn their profits.
In this article, we will see on how AI price optimization works, advantages, How to implement , and some examples.
The Power of AI Price Optimization
AI price optimization means dynamically changing the price for a given service based on the customer’s behavioral and statistical data received from the system all in real time, theyby increasing the profit margin.
This method is different from the traditional ways of changing the price ie. manually going in the system to change the price of a service based on the input data. This uses real time data and conditions set by the service owner.
These method not only maximizes revenue but also maintains the customer satisfaction (CSAT) score.
Benefits of AI Price Optimization
Implementing dynamic pricing into your system involves several steps. These are :
- Data Collection
- The primary step is to collect the data and aggregate the needed data needed for price prediction. These data may include your system’s historical pricing data, your competitors data for the specific service, identifying the demand in the market and the current trend based on the geographical region for that specific service.
- The more data system has access to, the more accurate will be the pricing prediction model.
- Data Analysis:
- Based on the collected data, these data now will be analyzed to identify the patterns and the trends for the specific service being offered to the customer.
- AI algorithms can process large amount of data very swiftly and accurate, and identify the correlation with data that would be missed by a human analyst.
- Algorithm Development
- The next step is to develop the AI model and train the AI on the collected data. An appropriate machine learning model should be selected to collect and train model on the collected data.
- The algorithm should be capable on predicting the real time demand in the market, identify the threshold price for the maximing the revenue, and adjust the prices accordingly for the specific service that benefits both the system owner and the customer.
- Integeration with existing systems
- These AI price optimization model needs to be implemented into the current transportation or logistics system. Also ensuring the price optimization model is seamlessly integerated across all the touchpoints for effective display and prediction.
- Continous Monitoring & Improvement
- The dynamic pricing model is not a implement and forget it solution. But a continous monitoring of the solution is needed that helps the AI model to improve and better equipped for unknown circumstances. Thereby, reducing the chance of unknown errors.
Real-World Examples
Several companies in the industry of transport and logistics have successfully implemented AI price optimization (dynamic pricing model) for maximizing their revenue.
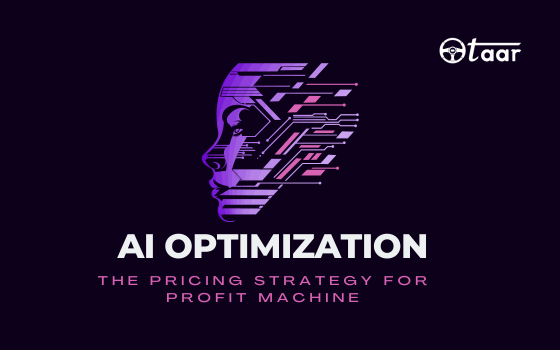
Tokyo Taxi
The large product tagline
Lorem ipsum dolor sit amet, consectetur adipiscing elit.
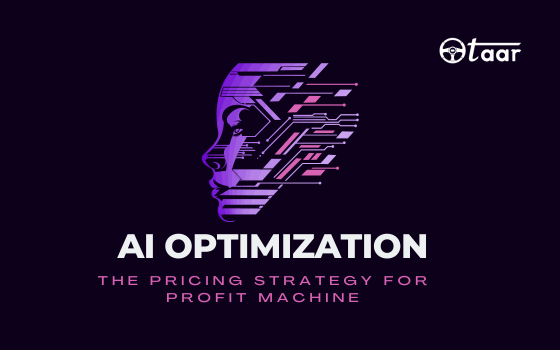
Tokyo Taxi
The large product tagline
Lorem ipsum dolor sit amet, consectetur adipiscing elit.
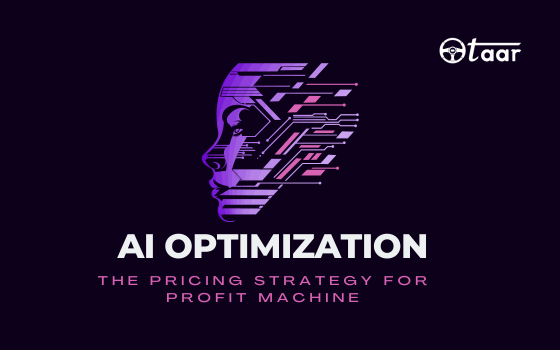
Tokyo Taxi
The large product tagline
Lorem ipsum dolor sit amet, consectetur adipiscing elit.
Example 1: Dynamic Pricing in Ride-Sharing Services
The well-known companies like Uber and Lyft use an AI price optimization model to adjust the prices in real time based on the real-time demand in that specific georegion. That helps them in two aspects, i.e., maximize their booking and also increase their revenue while staying on par with the driver share ratio.
You might have well seen during peak hours or in the high-demand areas, the surge pricing changes in real time to meet the market demands and also ensure they get the maximum reservations.
These dynamic pricing models have been very beneficial to these companies to maximize their profit and still offer a competitive fare.
Example 2: Freight & Logistics Optimization
Companies like DHL, FedEx, and others use the AI dynamic pricing model to manage their shipping rates. They analyze multiple factors such as fuel cost, delivery time and location, current demand in that specific georegion, and competitor pricing. These models help them increase their revenue while maintaining customer satisfaction.
Example 3: E-commerce delivery
Marketplace leaders such as Amazon and Instacart use AI price optimization models for their product delivery. By dynamically adjusting their delivery prices based on factors such as order size, delivery location, delivery time, and the existing number of orders in that specific georegion.
This has been a major beneficial factor for their market positioning that maximizes their revenue while still maintaining their customers satisfaction.
Challenges and Considerations
While the AI price optimization has great benefits, but it does come with several challenges. Companies need to consider several factors before proceeding with model implementation. These are :
- Data Quality : The quality of data used for the pricing prediction model is the oracle. Companies have to ensure their data quality is good and updated.
- Biased Algorithm : An AI algorithm can at times produce biased results. So it is highly important to review the produced results and adjust the algorithm accordingly if the results produced are biased.
- Compliance regulatory : The AI dynamic pricing model can at times raise regulatory concerns based on the geo region that it is served. So it is absolutely important, that all such compliances are regularly part of the pricing prediction model and continuously verified and validated.
- Percepetion of Customer : While the goal of AI dynamic pricing is to ensure profitability, but it can lead to customer dissatisfaction at times. Companies need to ensure that their pricing strategy based on the model trained is in line with customer’s value purchase and be transparent.
Future Trends in AI Price Optimization
The future of AI dynamic price optimization model looks very promising from where we are today. Several trends that would shape the transport and logistics industry in the coming years would be:
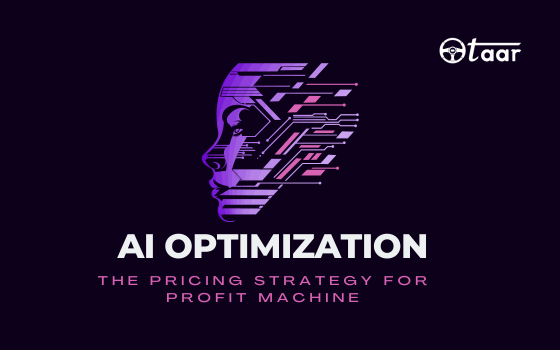
Tokyo Taxi
The large product tagline
Lorem ipsum dolor sit amet, consectetur adipiscing elit.
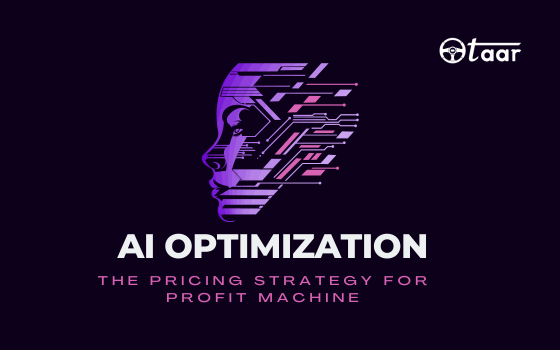
Tokyo Taxi
The large product tagline
Lorem ipsum dolor sit amet, consectetur adipiscing elit.
Integeration with IoT:
The integeration of AI price optimization model with IoT (Internet of things) devices would account to more accurate pricing, based on real time data received from these devices. These devices have the capability to produce real time based on factors such as traffic conditions, weather, vehicle performance that will enhance the pricing algorithm.
Advanced predictive analytics:
The future development in predictive analytics will help even more accurate pricing and demand forecasting. It would help companies to predict the market trends and adjust the service pricing.
Personalized pricing:
The AI price optimization model will give more superhero powers to the companies to offer personalized pricing to their customer based on the customers behavioral data and their individual preferances. Ensuring customer satisfaction and loyalty.
Blockchain integeration:
The blockchain model will further ensure the transparent pricing and security of the AI price driven models. This would help build the trust with customers while maintaining compliances with regulatory bodies all the time.
Conclusion
The AI price optimization model can be a powerful tool for the new age transport and logistics companies that would help in maximizing their profits while remaining competitve.
By leveraging AI and real time data, companies can auto adjust the service pricing, and ensure customer satisfaction, and improve their operational efficiency.
While challenges are there, but the challenges in the current market outweigh the benefits associated with new technology. And with time, it would be achieve near perfection.
With the rise in technology, things are only going to get better. The advantage is definitely for the first mover that would define competitve edge and win their customers trust.